numl alternatives and similar packages
Based on the "Machine Learning and Data Science" category.
Alternatively, view numl alternatives based on common mentions on social networks and blogs.
-
Accord.NET
DISCONTINUED. Machine learning framework combined with audio and image processing libraries (computer vision, computer audition, signal processing and statistics). -
TensorFlow.NET
.NET Standard bindings for Google's TensorFlow for developing, training and deploying Machine Learning models in C# and F#. -
m2cgen
Transform ML models into a native code (Java, C, Python, Go, JavaScript, Visual Basic, C#, R, PowerShell, PHP, Dart, Haskell, Ruby, F#, Rust) with zero dependencies -
AForge.NET
AForge.NET Framework is a C# framework designed for developers and researchers in the fields of Computer Vision and Artificial Intelligence - image processing, neural networks, genetic algorithms, machine learning, robotics, etc. -
Deedle
Easy to use .NET library for data and time series manipulation and for scientific programming -
Accord.NET Extensions
DISCONTINUED. Advanced image processing and computer vision algorithms made as fluent extensions. -
Catalyst
๐ Catalyst is a C# Natural Language Processing library built for speed. Inspired by spaCy's design, it brings pre-trained models, out-of-the box support for training word and document embeddings, and flexible entity recognition models. -
SciSharp STACK
A rich machine learning ecosystem for .NET created by porting the most popular Python libraries to C#.
InfluxDB - Power Real-Time Data Analytics at Scale
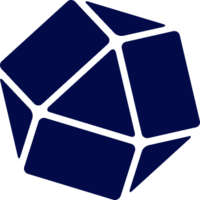
* Code Quality Rankings and insights are calculated and provided by Lumnify.
They vary from L1 to L5 with "L5" being the highest.
Do you think we are missing an alternative of numl or a related project?
README
Project Description
This library is designed to assist in the use of common Machine Learning Algorithms in conjunction with the .NET platform. It is designed to include the most popular supervised and unsupervised learning algorithms while minimizing the friction involved with creating the predictive models.
Contributing
I would love to take contributions! Please read this.
Learn More
More details can be found at the project website: http://numl.net.